In Silico, Machine Learning-Based Platform to Identify Disease-Causing Senescent Cells
Accurate and reproducible method to identify senescent cells for diagnostic and therapeutic applications.
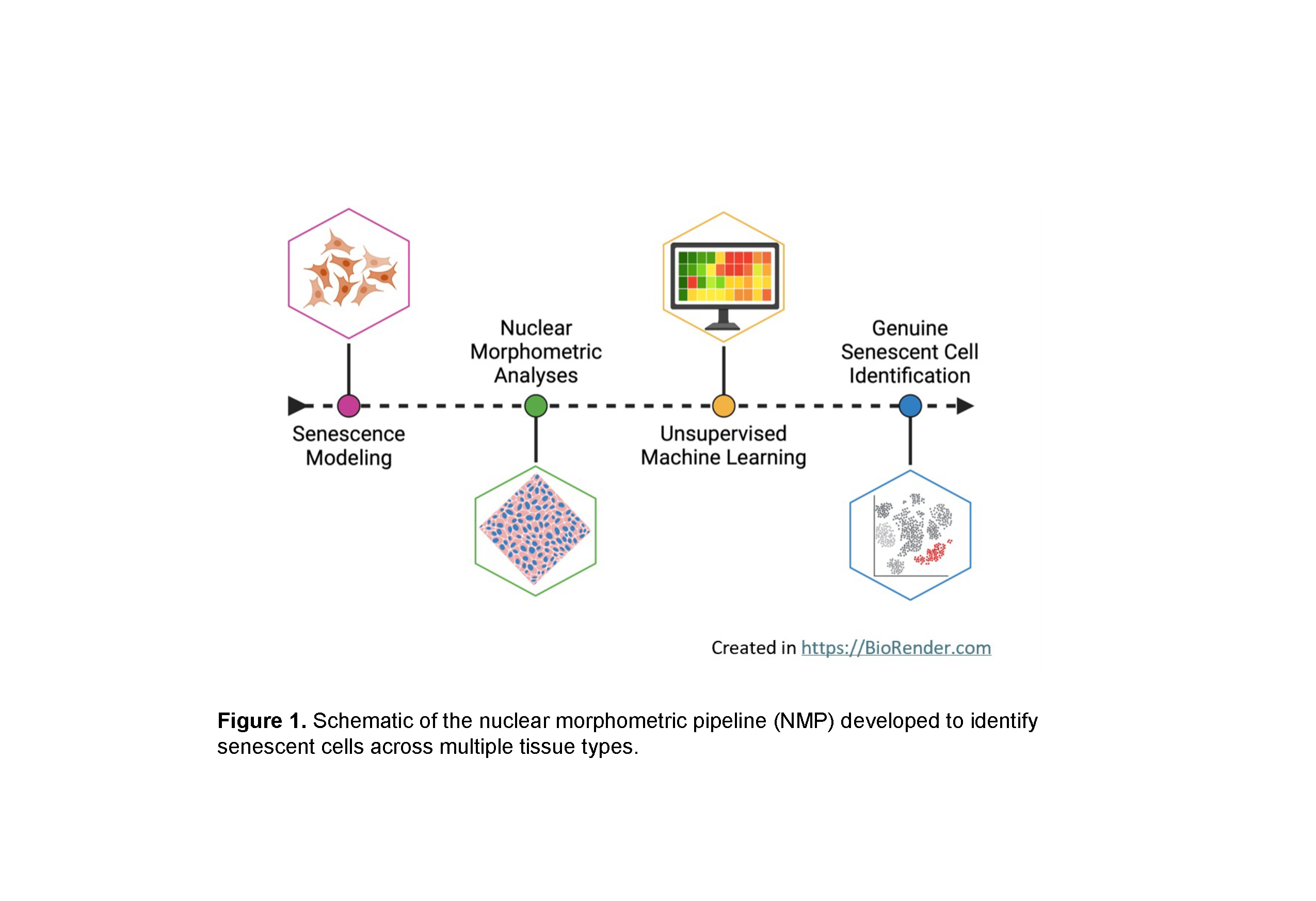
Technology
The NYU inventors have developed an efficient in silico pipeline, termed the “nuclear morphometric pipeline” (NMP), to rapidly and accurately identify senescent cells (SnCs) for diagnostic purposes and/or for therapeutic targeting in a variety of disease contexts. This robust pipeline (Figure 1.) was established by applying unsupervised machine learning algorithms to detect and quantify nuclear morphologies in skeletal muscle and cartilage, two tissues known to contain SnCs. Nuclear morphologies (size, circularity, foci and DAPI intensity) are dimensionally reduced and scored to produce a novel Senescence Score that can identify SnCs. This computational approach is extremely easy to practice, reproducible, and more accurate than commonly used biomarker-based approaches (e.g., SA-β-galactosidase). As described in unpublished proof-of-concept studies, the NYU inventors tested their NMP on normal and injured skeletal muscle tissue and showed temporal changes in the SnC environment based on age and injury progression. Additionally, to confirm the NMP’s utility for age-related degenerative disorders linked with SnC load, the same process was applied to a model of aged osteoarthritis (geriatric mice with degenerative articular cartilage). NMP analysis revealed a significant senescent signature in the chondrocytes of geriatric cartilage, indicated by the 10-fold increase in SnCs when compared to chondrocytes from young healthy tissue. In summary, this in silico platform offers an innovative method to reliably detect and score SnCs at single-cell resolution in a variety of normal and diseased tissue contexts, paving the way for the next generation of senolytic-related diagnostics and therapeutics.
Background
Senescence is a biological process by which cells limit their proliferation and secrete proinflammatory cytokines, chemokines, and growth factors, known as the senescence-associated secretory phenotype. Senescent cells accumulate as people age and contribute to chronic inflammation and fibrosis. Accurately identifying and effectively targeting senescent cells is a strategy that would be therapeutically relevant in a myriad of prevalent and debilitating age-related conditions and diseases, such as osteoporosis, certain cancers, chronic kidney disease, and neurodegeneration. Commonly employed biomarker approaches for identifying senescent cells (SnCs) face challenges in accurate detection due to cell heterogeneity and context-specific phenotypes. Moreover, current biomarkers (p16, p21, HMGB1, SA-β-gal, and LaminB1) show significant variability across different studies and detection assays. Development of next-generation senolytic therapeutics is similarly encumbered by the difficulties in identifying senescent cells. Currently approved senolytic molecules, such as Dasatinib and Quercitin, are non-specific and induce significant off-target toxicity, reducing their therapeutic indexes substantially. Consequently, there is an unmet need to develop improved methodologies for accurate and reproducible SnC detection for diagnostic and therapeutic targeting applications.
Development Status
The inventors have demonstrated that NMP morphological measures are conserved across cell types, tissue contexts, and mechanisms of senescence induction. The inventors now plan to deploy the NMP to: 1) identify genuine SnCs as a biomarker for biological organ age; 2) assess senolytic efficiency in targeting genuine SnCs; and 3) reveal cell-specific druggable mechanisms for SnCs.
Applications
Diagnostic and therapeutic targeting applications, including:
- age-related lipodystrophy,
- cancer, sarcopenia,
- muscular dystrophy,
- neurodegenerative diseases,
- renal dysfunction,
- osteoporosis,
- osteoarthritis,
- COPD,
- cirrhosis,
- cataracts,
- atherosclerosis,
- idiopathic pulmonary fibrosis,
- premature aging,
- and other age-related disorders.
Advantages
- Rapid and cost-effective methodology: Senescence scores can be generated in 24 hours from sample imaging to the score readout. If microscopes and computer workstations are in place, the cost will be <$100 for reagents like DAPI and PBS.
- Consistent and reproducible analysis: Consistent performance across different tissue types due to nuclear morphometric-based analysis.
- High accuracy and applicability across multiple cell types: For example, stem cells, fibroadipogenic progenitors, endothelial cells, skeletal muscle cells, chondrocytes, immune cells, myoblasts, adipocytes, and epithelial cells.
Intellectual Property
NYU has filed a U.S. provisional patent application covering the workflow, senescence score calculation, and the method of using the workflow and the score to detect senescent cells for the diagnosis and/or treatment of disease.